How to Learn Anything (in Climate)
A Practical Guide to Learning Frontier Knowledge Like a Nobel Laureate
👋 Hej to 2,796 climate buddies 🌳
Climate is not a technology problem but a story problem.
Delphi Zero is a consultancy and newsletter dealing with the exciting and beautiful world of climate tech.
📧 If you are opening this essay in your email inbox, I recommend to click on the title of this piece to enjoy the full-length version in the browser.
Dear friendos, this is a quasi-follow up to my previous essay “How the CIA Would Evaluate Climate Tech.” Both essays have been inspired by spit-balling ideas with other climate brains at the Drop conference.
Today’s essay is about the learning framework that Ben and I taught at the conference.
This is your personal 10-minute crash course in effective learning 👇
How to Learn Anything (in Climate)
By Art Lapinsch
The Great Explainer's Secret Sauce(s)
Richard Feynman is an intellectual crush of mine:
1965 Nobel Prize-winner for his work in quantum mechanics
Dubbed “The Great Explainer” for his ability to explain complex topics with simple words
And one of the people who turned the Manhattan Project into the history-defining endeavor we all know
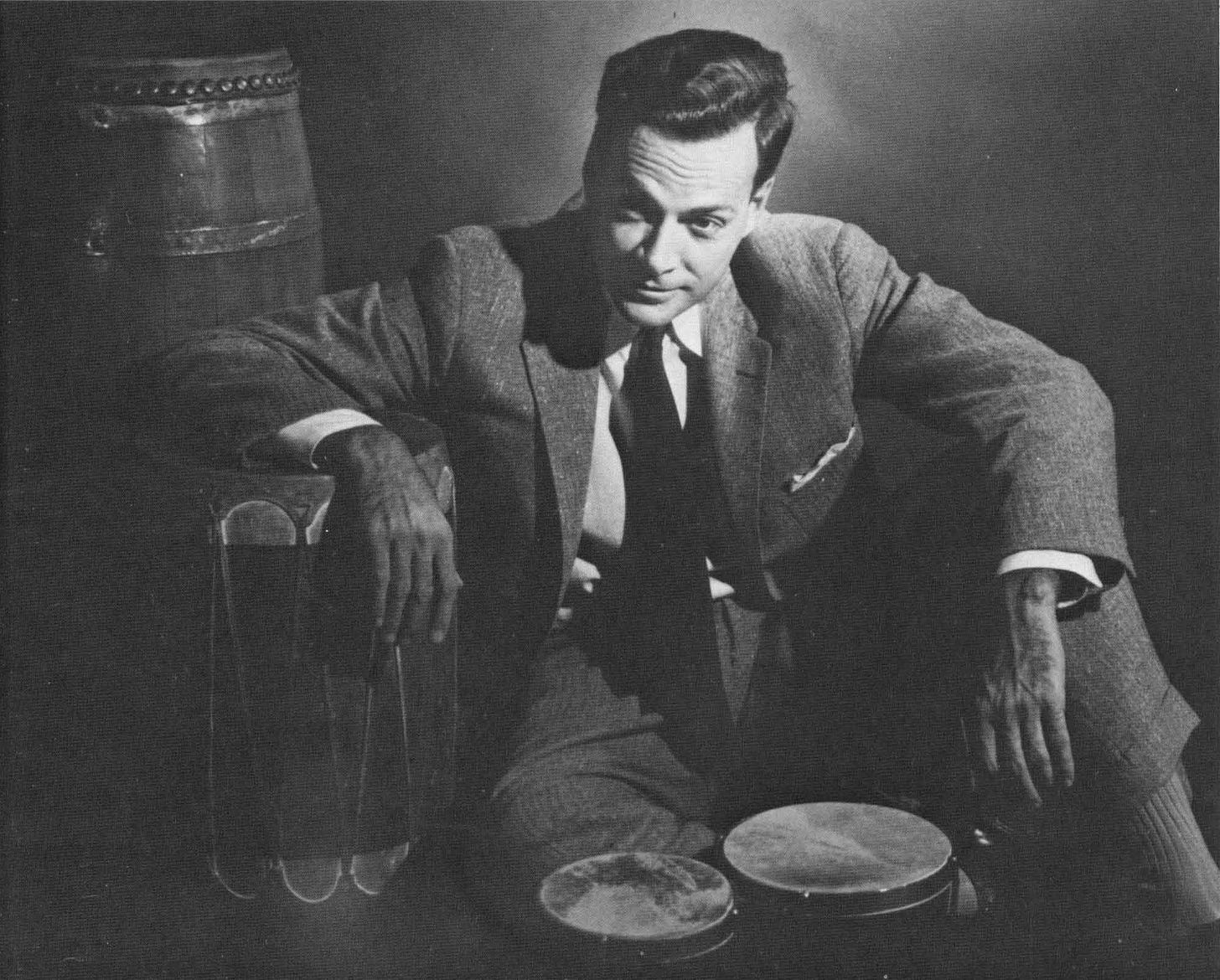
Feynman’s Signature Sauce: Teach to Learn
If you know Feynman, then you might be familiar with his didactic maxim:
“If you want to master something, teach it.”
He reasoned that in order to convey something clearly to an audience, you must truly understand it. By teaching it, you’ll quickly uncover the gaps in your own understanding.
The method can be mapped as follows: Choose a concept → teach it → identify gaps → review and simplify → rinse and repeat.
But this only applies if we already know about a subject.
So to all the scholars and scientists out there: Try teaching your knowledge to someone else. You might learn a thing or two.
But - for the rest of us - the question remains: How do we learn something new?
This is where Feynman’s lesser known technique comes into play 👇
Feynman’s Secret Sauce: What → Why → How
Hidden in the Feynman Lectures at CalTech, there’s a golden nugget.
Feynman argues that teaching/learning should follow this sequence:
Who: The learner 🤷 → What’s their context? What do they know already? What’s the language that they speak and understand? etc.
What: The topic ⛳️ → What needs to be learned? What’s important? What’s not? What are the boundaries of the content? What’s out of scope? etc.
Why: The purpose 💚 → What are the practical implications of the acquired knowledge? Why bother? Why does it matter to the learner? etc.
How: The method 🗺️ → What is the best method to teach the topic under the given circumstances (who/what/why)?
I’ll give you a practical example that blew my little mind.
Feynman’s Los Alamos Episode: Learning Advanced Nuclear Physics from Scratch
When Feynman moved to Los Alamos to join the Manhattan Project, he was a PhD from Princeton University but his expertise was primarily in quantum mechanics and quantum electrodynamics.
In case someone didn’t know, the purpose of the Manhattan Project was to build an atomic bomb. So naturally, fundamental and advanced knowledge of nuclear physics was crucial. But Feynman didn’t know advanced nuclear physics (yet).
The Manhattan Project hired someone to build an atomic bomb without being an expert in atomic physics. What the crap!
In his autobiography “Surely You're Joking, Mr. Feynman!” he wrote about this episode. If we plug it into our little framework, then we see this:
Who: Richard Feynman, PhD 👨🏫 → One of the world’s leading physicists and researchers in quantum physics.
What: Nuclear Physics ⚛️ → Nuclear fission, chain reactions, neutron diffusion, etc.
Why: Win the War ⚔️ → It was believed that Nazi Germany was months ahead in developing their version of the atomic bomb. The stakes couldn’t be higher.
How: Lab Exposure 🗺️ → The fastest way he could learn the topics to work side-by-side with the leading nuclear physicists and researchers. It’s faster to ask Szilard, Bethe, or Lawrence than it is to find it in a physics book. Why? It wasn’t commonly documented knowledge (yet).
His How of acquiring this knowledge was a result of his context.
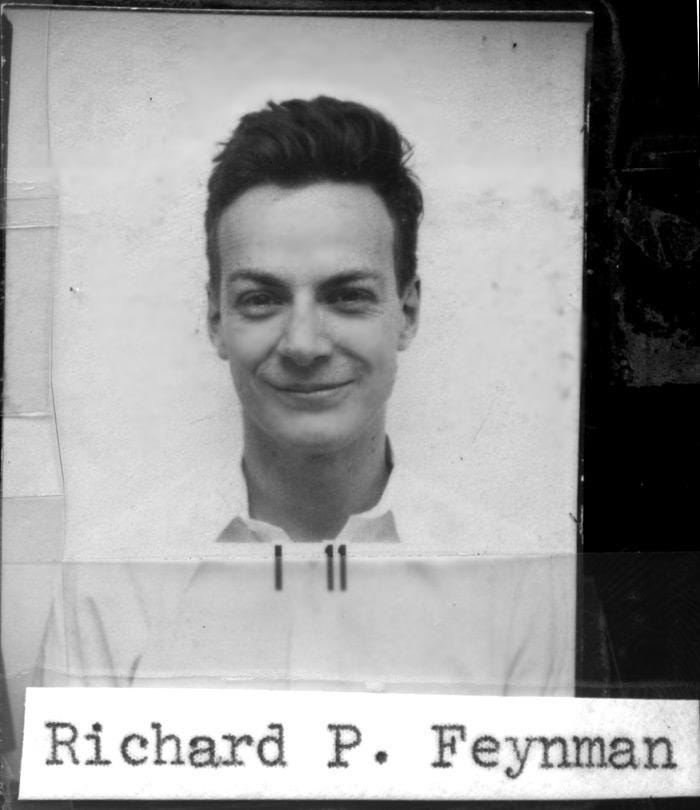
Personal Case Study: Learning About New Markets
I’ll give you another practical example from my own life: Learning about data centers.
Recently, I started feeling the entrepreneurial itch again and started exploring a couple of problem/trend spaces:
etc.
I’ll show you how I approached my data center x energy research 👇
Using Feynman’s Secret Sauce
My situation could be mapped as follows:
Who: Art Lapinsch ✌️ → Former AdTech founder w/ degree in Energy Law and understanding of electricity market regulation
What: Energy Demand for Data Centers ⚡️ → Scope; Growth rates; Road Blocks; etc.
Why: Understand the Market 💡 → The map; the players; the flows; etc. (see above 👆)
How: In-depth Content 🗺️ → Many hours of podcast conversations about various topics related to data centers; detailed blog posts; etc.
My What/Why-combo informs the How.
When I’m trying to understand a market, I usually follow this process that I shared in a previous essay:
My first goal was to build a mental map of the
climatedata center space:Where are the edges of the map?
Who is participating?
How do the various groups interact?
What are the main flows in this world?
Which fundamental knowledge do I need to learn?
What are common misconceptions?
The goal in this step is to get enough of an understanding to start having conversations with domain experts. This is my “desk research” so to speak.
My InternetU Curriculum - Written Content
To get a first grasp of the problem, I would google and read as much as I can:
AI will use a lot of energy. That's good for the climate.: A high-level case for the positive economic impacts of data center expansion.
Free Guide to Data Center Power: A technical resource with lots of figures and illustrations.
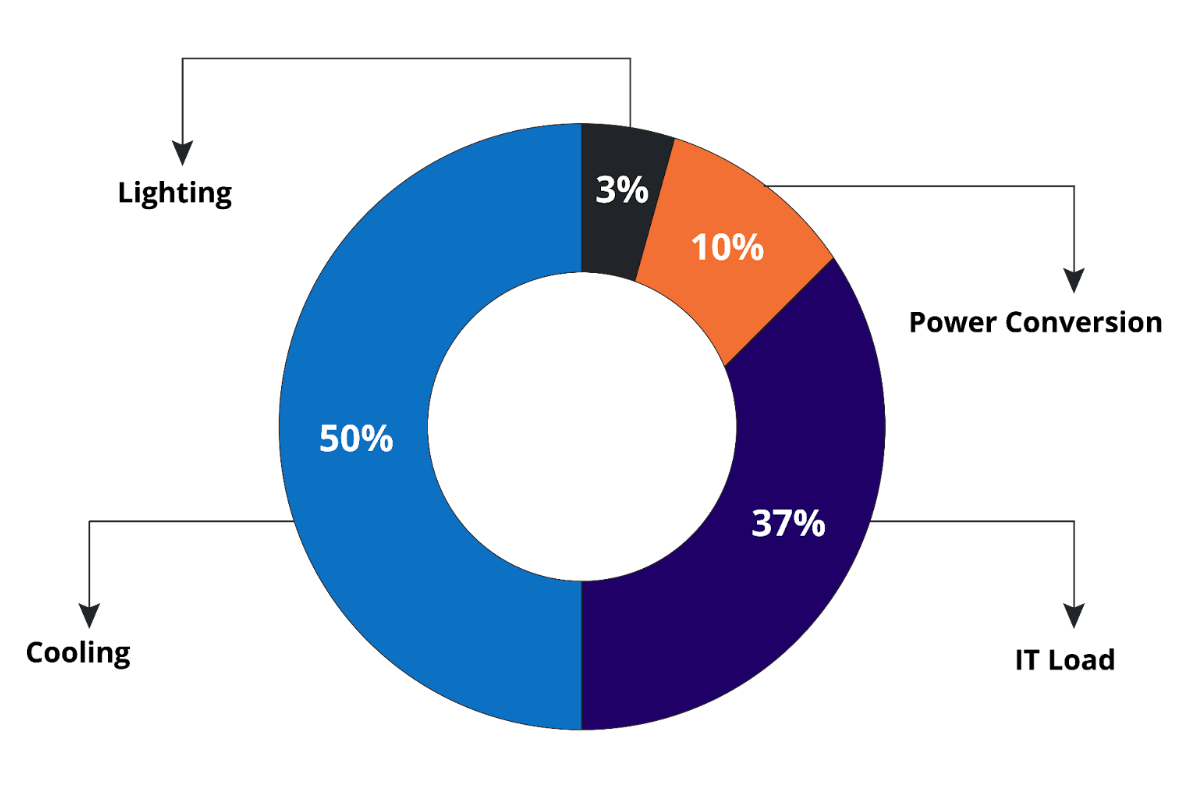
A Roadmap for Sustainability in AI: A practitioners view on data center’s ESG impact + possible fixes.
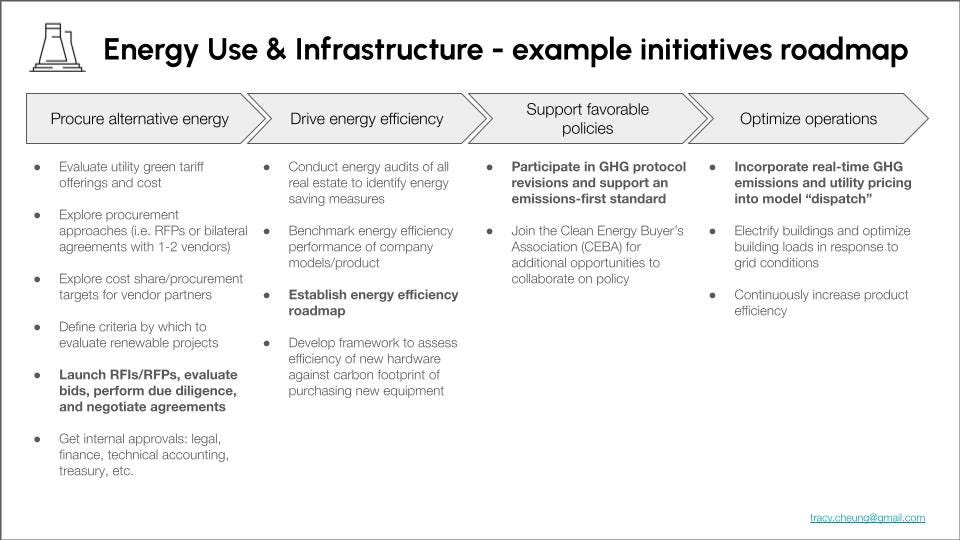
Steel, Servers and Power: What it Takes to Win the Next Phase of AI: Nice lens on project development/financing.
Here’s how it works behind the scenes:
A real estate developer—QTS, Vantage and CyrusOne are three popular ones—goes and buys land and power that they believe a data center can be built on.
The developer approaches the big tech companies and offers them a 15-year or 20-year lease on the data center, for a total cost of $2-10B.
Once the developer has a signed deal, they go to the capital markets, and they raise debt against the deal, usually from banks or real estate investors.
The debt investors are not underwriting the future AI demand in the data center—they are underwriting the credit of the customer, say Microsoft or Amazon, and expect a yield that is a slight premium to corporate debt.
The developer goes and hires a general contractor—for example, DPR, one of the most popular data center builders.
The general contractor goes and hires subcontractors. The subcontractors then go and recruit labor. Labor is a huge component of the cost of data center construction.
Labor moves to the location where the data center is getting built—for example, a small town or city—and they are put up in hotels or other accommodations in the area.
Over a two year period, a massive construction project takes place, starting with steel and concrete for the overall structure, and ending with industrial parts and GPUs being installed.
During this whole process, the end-user of the data center (e.g., Microsoft or Amazon) is negotiating with their own supply chain for diesel generators, liquid cooling systems, and other necessary equipment.
After reading all of this, I’m already a bit more informed than before.
My InternetU Curriculum - Audio Content
After learning some of the abbreviations, players, and concepts, I feel equipped enough to consume hour-long podcasts.
My favorites so far:
The Complete History & Strategy of Amazon Web Services: Helps to get a grasp of the scale and stickiness of data center business models.
Nvidia Part III: The Dawn of the AI Era (2022-2023): Helps to understand (a) what is meant when people talk about “the data center becomes the computer” and (b) why bandwidth and networking technology is a major bottleneck in data centers.
Not Boring Founders: Crusoe Energy (Chase Lochmiller and Cully Cavness): Grasp why it is “easier to move data than it is to move gas fields.”
Saving the Planet with Better AI Data Centers (with Crusoe CEO Chase Lochmiller): Deep-dive into building modular data centers that are co-located next to oil wells.
Use Feynman’s Secret Sauce: What → Why → How
If Feynman can learn nuclear physics at Los Alamos, you can learn X in Y.
The key to structuring your own learning is to understand the who/what/why before you go all-in on the how. Different things work for different people and that’s ok.
My intuition tells me that a lot of the exciting climate tech is frontier stuff. There are no lectures or HBS Case Studies on it (yet). But there are enough operators who write and speak about it on the internet. Your task is to find the golden nuggets in the haystack of the internet.
So the next time you try to learn some frontier knowledge, ask yourself:
Who: The learner 🤷 → What’s their context? What do they know already? What’s the language that they speak and understand? etc.
What: The topic ⛳️ → What needs to be learned? What’s important? What’s not? What are the boundaries of the content? What’s out of scope? etc.
Why: The purpose 💚 → What are the practical implications of the acquired knowledge? Why bother? Why does it matter to the learner? etc.
How: The method 🗺️ → What is the best method to teach the topic under the given circumstances (who/what/why)?
Chances are that you will find something good.
🙏 Thanks Ben and Nick for spit balling about data centers.
I'd love to hear about your experiences with the Feynman Method or other learning strategies. Feel free to respond to this email or leave a comment below.
Share this essay with someone who's eager to dive deeper into climate tech learning.
Richard Feynman 🫶 my intellectual crush. He's always my answer to the "if there was one person you would like to have a dinner with, who.."